MongoDB Atlas Analytics with Amazon SageMaker on AWS
Partner Solution Deployment Guide
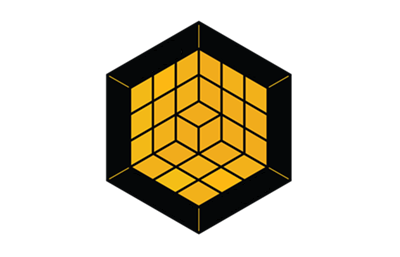
April 2023
Zuhair Ahmed, MongoDB Inc.
Vinod Shukla, AWS Integration & Automation team

Refer to the GitHub repository to view source files, report bugs, submit feature ideas, and post feedback about this Partner Solution. To comment on the documentation, refer to Feedback. |
This Partner Solution was created by MongoDB Inc. in collaboration with Amazon Web Services (AWS). Partner Solutions are automated reference deployments that help people deploy popular technologies on AWS according to AWS best practices. If you’re unfamiliar with AWS Partner Solutions, refer to the AWS Partner Solution General Information Guide.
Overview
This Partner Solution deploys a MongoDB Atlas cluster with Amazon EventBridge and Amazon SageMaker. It helps you start working quickly with your machine learning (ML) models using MongoDB as data source and Amazon SageMaker for data analytics.
Costs and licenses
There is no cost to use this Partner Solution, but you will be billed for any AWS services or resources that this Partner Solution deploys. For more information, refer to the AWS Partner Solution General Information Guide.
This Partner Solution deploys MongoDB Atlas resources with the latest stable MongoDB enterprise version, which is licensed and distributed under the Server Side Public License (SSPL).
Architecture
Deploying this Partner Solution with valid parameters builds the following environment in the AWS Cloud.

As shown in Figure 1, this Partner Solution sets up the following:
-
In the MongoDB SaaS account:
-
A MongoDB cluster.
-
A MongoDB Atlas Realm trigger to send database change events to Amazon EventBridge in the customer account.
-
-
In the customer account:
-
EventBridge to ingest MongoDB change events from the Realm trigger and send SageMaker result events back to the MongoDB database. EventBridge uses the following event buses and rules:
-
A Partner SaaS event bus to receive MongoDB change events from the Realm trigger.
-
A rule to route events to the pull events AWS Lambda function.
-
A custom event bus to receive SageMaker result events from the pull events Lambda function.
-
A rule to route results to the push results Lambda function.
-
-
Two Lambda functions:
-
A pull events function to read events from the Partner SaaS event bus and get results from SageMaker.
-
A push results function to write results from SageMaker to the MongoDB database in the MongoDB SaaS account.
-
-
An Amazon Simple Storage Service (Amazon S3) bucket for model-training artifacts.
-
Amazon SageMaker to provide an endpoint to a pretrained ML model.
-
Deployment options
This Partner Solution provides the following deployment options:
-
Deploy MongoDB Atlas Analytics with Amazon SageMaker. This option deploys the MongoDB Atlas Analytics with Amazon SageMaker Partner Solution as described in the Architecture section, earlier in this guide.
Predeployment steps
Prepare your AWS Account
This Partner Solution uses MongoDB Atlas CloudFormation resource types and automatically registers the MongoDB::Atlas::Trigger
resource in the AWS Region of your choice. Once it’s running, you can safely skip this step for additional deployments in each Region by setting the ActivateMongoDBTriggerResource
parameter to No.
Prepare your MongoDB Atlas account
A MongoDB Atlas programmatic API key must be generated with the appropriate permissions and network access entries so that AWS CloudFormation can successfully authenticate the MongoDB cloud. For more information about creating and managing API keys, refer to Get Started with the Atlas Administration API.
Create a MongoDB Atlas cluster
Create a MongoDB Atlas cluster, either using your MongoDB Atlas account or the MongoDB Atlas on AWS Partner Solution.
Create Realm app and service
A MongoDB Realm app and service to create triggers that send events to AWS EventBridge for further processing. For more information, refer to the following:
Use the access token as a bearer token in the Authorization header in the further calls.
|
Keep the Application ID and Service ID handy for further steps. |
Upload ML model artifacts to Amazon S3
Upload your ML model artifacts to Amazon S3. Create an AWS managed Deep Learning Container Image or a custom image in Amazon Elastic Container Registry (Amazon ECR) to deploy and run the model. For more information, refer to the sample code provided in the mongodb/mongodbatlas-cloudformation-resources GitHub repository.
Create Amazon ECR images for pull and push Lambda functions
Create an Amazon ECR container image for the pull Lambda function that reads MongoDB events from EventBridge and the push function that writes results back to MongoDB. For more information, see Creating Lambda container images.
Deployment steps
-
Sign in to your AWS account, and launch this Partner Solution, as described under Deployment options. The AWS CloudFormation console opens with a prepopulated template.
-
Choose the correct AWS Region, and then choose Next.
-
On the Create stack page, keep the default setting for the template URL, and then choose Next.
-
On the Specify stack details page, change the stack name if needed. Review the parameters for the template. Provide values for the parameters that require input. For all other parameters, review the default settings and customize them as necessary. When you finish reviewing and customizing the parameters, choose Next.
Unless you’re customizing the Partner Solution templates or are instructed otherwise in this guide’s Predeployment section, don’t change the default settings for the following parameters: QSS3BucketName
,QSS3BucketRegion
, andQSS3KeyPrefix
. Changing the values of these parameters will modify code references that point to the Amazon Simple Storage Service (Amazon S3) bucket name and key prefix. For more information, refer to the AWS Partner Solutions Contributor’s Guide. -
On the Configure stack options page, you can specify tags (key-value pairs) for resources in your stack and set advanced options. When you finish, choose Next.
-
On the Review page, review and confirm the template settings. Under Capabilities, select all of the check boxes to acknowledge that the template creates AWS Identity and Access Management (IAM) resources that might require the ability to automatically expand macros.
-
Choose Create stack. The stack takes about 10-20 minutes to deploy.
-
Monitor the stack’s status, and when the status is CREATE_COMPLETE, the MongoDB Atlas Analytics with Amazon SageMaker deployment is ready.
-
To view the created resources, choose the Outputs tab.
Postdeployment steps
Test the deployment
To test the solution, insert data into the MongoDB collection, either manually or using an application. For more information, refer to Collections and Insert Documents.
Troubleshooting
For troubleshooting common Partner Solution issues, refer to the AWS Partner Solution General Information Guide and Troubleshooting CloudFormation.
Errors can typically be resolved by inspecting CloudFormation event logs or CloudWatch logs created by the MongoDB Atlas CloudFormation resources.
If a stack fails to deploy, check the Events tab. If the error occurs for one of the MongoDB Atlas resources (for example, MongoDB::Atlas::Trigger
), locate the corresponding Amazon CloudWatch Logs group called mongodb-atlas-trigger-logs. Check the latest log entry to identify the issue. Additionally, verify that the parameter inputs are valid and that all of the Amazon ECR image URIs are in the same AWS Region, and check the error messages for each failed resource.
If the pull or push Lambda functions are unable to process events, check if the event rule has the proper event patterns. Update the event rules according to your requirements.
If Amazon SageMaker results are inappropriate, verify that all of the model artifacts are uploaded to Amazon S3 and that the Amazon S3 link is valid. Update the inference filename in the template if filename is not inference.py
.
Customer responsibility
After you deploy a Partner Solution, confirm that your resources and services are updated and configured—including any required patches—to meet your security and other needs. For more information, refer to the Shared Responsibility Model.
Feedback
To submit feature ideas and report bugs, use the Issues section of the GitHub repository for this Partner Solution. To submit code, refer to the Partner Solution Contributor’s Guide. To submit feedback on this deployment guide, use the following GitHub links:
Notices
This document is provided for informational purposes only. It represents current AWS product offerings and practices as of the date of issue of this document, which are subject to change without notice. Customers are responsible for making their own independent assessment of the information in this document and any use of AWS products or services, each of which is provided "as is" without warranty of any kind, whether expressed or implied. This document does not create any warranties, representations, contractual commitments, conditions, or assurances from AWS, its affiliates, suppliers, or licensors. The responsibilities and liabilities of AWS to its customers are controlled by AWS agreements, and this document is not part of, nor does it modify, any agreement between AWS and its customers.
The software included with this paper is licensed under the Apache License, version 2.0 (the "License"). You may not use this file except in compliance with the License. A copy of the License is located at https://aws.amazon.com/apache2.0/ or in the accompanying "license" file. This code is distributed on an "as is" basis, without warranties or conditions of any kind, either expressed or implied. Refer to the License for specific language governing permissions and limitations.